Related Content
Case Study
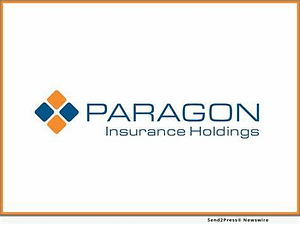
Case Study: Paragon Insurance Holdings
Paragon selected LeapFrogBI to build a new data warehouse and implement a more flexible business intelligence solution.
Resources
Enabling Advanced Insurance Analytics and Reporting
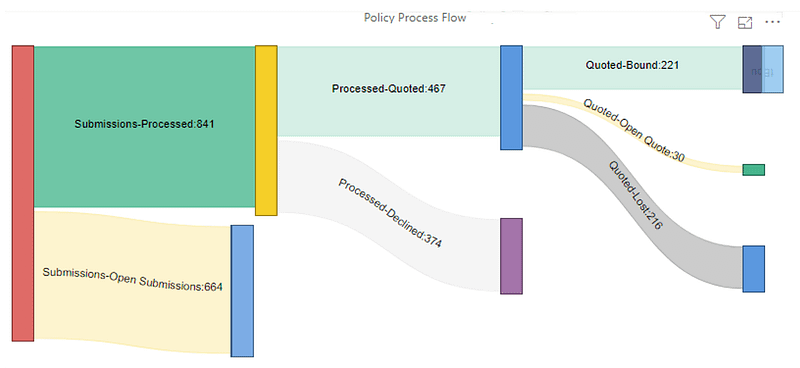
Enabling advanced insurance analytics is both challenging and highly rewarding. This article explains the challenge, recommends a proven solution and shows specific examples.
Advanced insurance analytics and reporting will lead to better decision making
Analytics are nothing new to the insurance industry. Successful insurance carriers long ago mastered the use of statistical analysis to assess the probability of loss, and price their risk accordingly. Ironically, employees at companies from agencies to carriers to third party administrators still lack the information necessary to be data-driven and optimize their business.Advanced insurance analytics and reporting can be a confusing topic. But the underlying concept is easy to grasp. It is the ability to give employees, at all levels of the organization, the information they need to make fact-based decisions in real time. With advanced analytics in place, employees can ask questions of data and understand the impact of their actions on performance. Best of all, they can do so without the need to involve technical resources.
The key performance indicators (KPIs) may vary depending on the type of company and the individual’s role, but the competitive advantage conferred by advanced reporting solutions is consistent across them all. This ability, to make fact based decisions in the moment, is a hallmark of successful companies in every industry. A global CFO study conducted by IBM showed that companies utilizing advanced analytics to drive decisions had twice the revenue growth and 20 times the EBITDA of their competitors.
By exposing data to business stakeholders, advanced reporting solutions quickly shine a light on data quality, business process and performance issues. This allows managers to identify missed opportunities and specific areas of improvement they can control. As they implement course corrections this process can dramatically improve the bottom line.
Insurance companies are no exception. If you want to outperform your competition, it is critical that you implement advanced analytics and reporting in your organization. Unfortunately, there are many ways this effort can go wrong, and only a few ways it can go right. Individual careers and entire companies hang in the balance, so it is worth taking a moment to explain the problem in some detail before we discuss the various solutions available.
We’re here to help and always glad to assist any way we can. Feel free to reach out if we may be of assistance.
Why is reporting so difficult?
All companies face challenges with analytics and reporting at one time or another. Though it may not be immediately obvious, all of these challenges stem from the same root cause — the necessary data exists, but isn’t easy to access or consume.
Modern reporting tools make data easy to visualize, but the resulting reports are only as good as the data feeding them. Before it can be utilized, we have to take all that data from internal systems, partners and 3rd-party data sources, and synthesize it into something useful. Data may need to be moved, integrated, aggregated, grouped, filtered or cleansed before it can be used to produce accurate and meaningful analytics. As the size and complexity of the data grows, and reporting needs become more advanced, the problem only gets worse.
The lack of “report-ready” data explains why data analysts, on average, spend 80% of their time preparing and organizing data. Anyone who has attempted to take raw data and calculate a loss ratio by accident year, or just about any advanced insurance analytics, will immediately understand the nature of the problem.
To learn what data visualization tools can and can’t do…
READ THIS:
Given the complexity of transforming data into advanced analytics, and the associated expense, choosing the best approach is critically important. At LeapFrogBI we specialize in the centralized approach, often referred to as data warehousing and business intelligence. This approach is ideal for producing dashboards and reports with KPIs that are needed on an ongoing basis.
How business intelligence will transform your company
There are many things to like about the centralized approach to advanced insurance analytics. It is the most cost-efficient approach, and it places advanced analytics and self-service reporting capabilities in the hands of the employees making decisions. This results in a data-driven culture, deeper engagement and increased strategic decision making. There a variety of other benefits, some technical and some business related.
The 7 Advantages of a Data Warehouse for Analytics and Reporting
Empowers everyone throughout the organization with the information they need to make correct decisions
Enables self-service analytics for diving deeper into the data
Illuminates data and business process issues that need improving
Sharing curated, validated data creates alignment across the organization
Data from all sources are integrated to provide a 360-degree view of the business
Reduces dependence on source systems and provides data retention
More cost-efficient than distributed approach to analytics
By contrast, the decentralized approach requires data transformation and other data science expertise throughout the organization. This comes at a huge cost, and often results in duplication of effort and different answers to the same questions. Of course, there are types of data analysis that the data warehouse can’t support, specifically predictive model development, segmentation model development and other types of extensive statistical analysis. These are perfect applications for point-solution data science, but it must be understood that distributed analytics is not a substitute for a centralized, governed data solution like a data warehouse when it comes to day-to-day reporting.
To better understand the data warehouse versus the data lake…
READ THIS:
What advanced insurance analytics look like in practice
Every insurance company will have a unique set of metrics to track based on the type of company and its unique focus. What follows are a few examples of insurance business intelligence KPIs you might see on an insurance dashboard. These particular metrics are related to production, underwriting, claims and other common insurance data.
Insurance KPIs Made Possible by a Data Warehouse and Business Intelligence
- Written Premium – The amount of premium written by policy effective date. Usually reported alongside forecasted written premium for the period. May be further segmented into new policies versus renewals, divided by line of business, etc.
- Earned Premium – The amount of premium for the elapsed period of each policy, calculated using the desired logic and business rules. Typically reported based on policy effective date and may be further segmented by coverage, line, program, risk state and other relevant dimensions.
- Commissions – The amount of money earned or paid as commissions by date. May be further segmented by coverage, line, program, employee/partner, etc.
- Distribution Performance – Policy counts, written premium and commissions by distribution channel partner by date.
- Rate Adequacy – Comparison of bound policy premiums to technical premiums by policy effective date. May be further segmented by coverage, line, program, underwriter, etc.
- Cycle Time – The number of days it takes a policy to progress from a submission to a bound policy. May be reported by period, underwriter, line, program, etc.
- Hit Ratios – The ratio of bound policies to submissions and bound policies to quotes. May be reported by time, line, program, underwriter, etc.
- Underwriting Process Efficiency – A visual depiction of policy flow showing status changes from submission to bound by quantity. (see an example)
- Loss Ratio – The ratio of incurred loss expense to earned premium, by program year, accident year and calendar year.
- Incurred and Reserve Amounts – by calendar year and by accident year, by program, line, etc.
- Policy Counts – New, renewal and endorsement transaction counts by time by program, line, coverage type, market segment, risk state, etc.
- Policy Renewal Retention Rate – Percent of expiring policies renewed, by period, cohort, program, line, etc.
Want to see and explore a sample insurance dashboard and reports?
SEE THIS:
Your preferred KPIs may look different, and the development process allows for that. When designing a data warehouse, each business unit and its stakeholders have the opportunity to participate by defining reporting requirements that dictate the data model and data transformation rules. You will also have the opportunity to define hierarchies in the data which will enable users to drill down to the most granular transaction details or roll results up to custom groupings you’ve defined.
For many people the concept of a data warehouse and business intelligence can be confusing. These terms have been used by different people to mean different things, so it is helpful to break this process down to basic concepts.
Every data problem requires a data solution
In the simplest terms, the data needed to generate advanced insurance analytics exists, but isn’t report-ready. To make it report-ready we write and automate processes that re-engineer the data nightly, or more frequently. The transformed data then becomes the new data source, and it is consumed using standard reporting and data visualization software.
It all starts with the data
Kerry Small, Vodafone
As stated by Kerry Small of Vodafone in HBR’s recent The New Decision Makers report, “It all starts with the data” and investing in a centralized data solution with a single record for everything “was the right thing to do.”
This data engineering process and its various components can take many forms. Choosing the best data solution depends on the number of data sources, data quantity, data quality, the complexity of reporting requirements and the existing data engineering and reporting infrastructure, if any.
Eventually, most insurance companies grow to the point where the benefits of a data warehouse outweigh the cost. The data warehouse has many advantages over other available data solutions, and chief among them is the dimensional data model.
The dimensional model is a unique data structure that was invented specifically to facilitate advanced reporting. When properly implemented, it allows non-technical users to ask very complex questions of the data with ease and receive answers in a fraction of a second. This concept of enabling self-service analytics is so powerful that we refer to it as the key to analytics that everyone should understand.
For a brief overview of available data solutions…
READ THIS:
Implementing an insurance data warehouse can be difficult. The overall failure rate for data warehouse projects is historically very high, and the costs are significant. There are important steps you can take to reduce, or even eliminate these risks.
7 Sure Fire Ways to Improve Data Warehouse Outcomes
- Commit to analytics as a business function
- Involve data warehouse experts early
- Include a persistent staging area (PSA)
- Use well-defined design patterns and code standards
- Load less data, not more
- Utilize agile instead of waterfall
- Leverage data warehouse automation
In a competitive and rapidly evolving market, Insurance companies that successfully enable fact-based decision making across the organization have a powerful advantage. But deciding to be great at analytics, and making it so, are two different things. Some of the work must be done in-house, such as developing a culture of information, investing in data governance and recognizing analytics as the critical business function that it is. Much of the work, however, is usually best left to expert partners with the specialized skills and tools necessary to succeed.